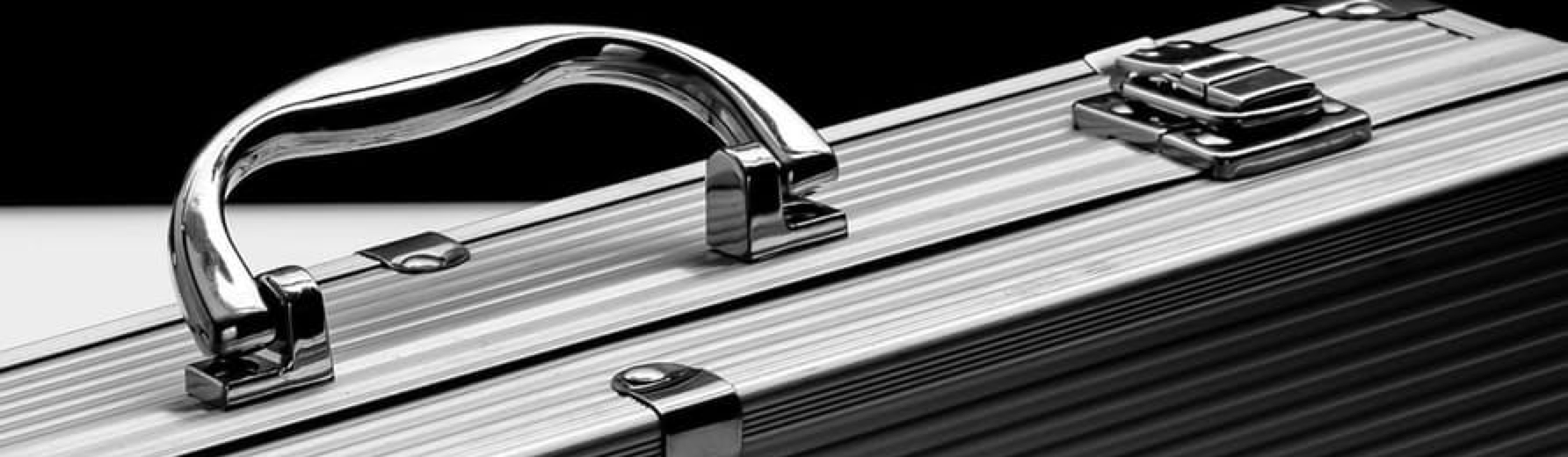
The need for data security is undeniable, and growing exponentially. According to Entrepreneur.com, by 2020, every person online will create roughly 1.7 megabytes of new data every second of every day, and that’s on top of the 44 zettabytes (or 44 trillion gigabytes) of data that will exist in the digital universe by that time.
Protecting data from the unwanted access and actions by unauthorized users—and from any other destructive force—is tantamount. And in most instances, the benefits of protecting the data far outweigh any negative consequences.
But this isn’t the case when it comes to the majority of analytics implementations today. Unfortunately, the very act of managing data security policies can prevent scale when it comes to analyzing data. And this can cause big problems for your business.
Data Security: Thwarting At-Scale Analytics
There are two common approaches for data analysis: downloading data to an endpoint device and then analyzing it, or analyzing data while it’s housed in a centralized data warehouse.
Let’s talk about the first approach. Some analytics solutions require users to download data to their devices before it can be analyzed. In addition to frustrating users (who must wait for what can be hours-long downloads to complete before analysis can begin), this approach breaches most companies’ data security policies. Once data downloads to a device, the enterprise has a hard time guaranteeing it isn’t shared in an unauthorized manner—all the more risky when that data is sensitive or confidential.
Alternatively, users analyze data while it remains safely stored on a central server. When dealing with larger data volumes, this approach historically required a data warehouse to act as the middleman between the central server and the user. And this presents a whole new host of challenges.
Take ERP. Companies spend millions of dollars and a ton of time implementing ERP solutions. And they spend much of that implementation time defining and putting in place data security rules—essentially, who can see what company data, when.
- Role-based permissions, for example, might let the CEO access all data, while department heads may only see data related to their area of ownership.
- Time-based permissions, for example, may restrict data access during quiet periods when employees and other insiders aren’t permitted to view preliminary results or discuss material company news.
In addition to their ERP system, these companies likely maintain several other core business systems from which data also needs to be pulled and analyzed in order to gain a complete understanding of the business. This is where the data warehouse comes in. It acts as the central repository for data coming from multiple core business systems and standardizes all formats, so analysis can be done in a uniform manner.
The catch is, when you move data from its original source to the data warehouse, you change the data format from relational to star schema (this transformation is referred to as “switching the original texture of the data”). Which means—and this is a big problem—you lose all the data security you spent time and money developing for the source system. Assuming you want to continue securing the data (which I’m sure you do), you have to re-implement security, which is a monumental undertaking. Even if you can complete the project, you cannot guarantee the data warehouse security policies will be exactly the same as the original security policies. And that’s a security risk in itself.
A second analytics challenge presented by data warehouse security is that companies typically only grant very few people access to data stored in the data warehouse. Many more people want to analyze data, and need to analyze it, but for data security reasons, only a few are allowed to actually do so. This constraint creates bottlenecks, delays and—in most cases—an incomplete or outdated view of company metrics.
So, what do you do? How do you choose between data security or information analysis when both are so critical to your business?
How Data Security and Analytics can Finally Co-Exist
The good news is, you don’t have to choose between them anymore. A new approach to analytics solves both dilemmas. It’s one of the driving reasons that led to my starting my company Incorta.
With our approach, you resolve the inherent conflict between data security and data analysis, so you can have both. Incorta:
- Centralizes data security with real-time access to corresponding data source security policies. We connect on-the-fly to the source systems, obtain all user credentials and then apply existing permissions to each user to automate which data they can access. There’s no downloading of data to endpoint devices. And, when you need to aggregate data from multiple systems (or add new systems to the mix), you can easily join the separate security policies and then apply them holistically to users.
- Delivers the fastest enterprise analytics. I’m talking “query-times-shrinking-from-hours-to-seconds” fast. In fact, we’re the only company that’s figured out how to maintain original security policies AND deliver queries faster than what you get by using a star-schema approach. This allows business users to have secure access to all business data, with faster performance than what they get with queries running on their desktop, with no data download required.
- Lets you easily scale analytics access to an unlimited number of users. You don’t need to restrict access to a handful of power users. Instead, you can give secure access to hundreds of users or even tens of thousands of users—whatever you need. That’s why a current Incorta customer is granting access to its analytics to a whopping 80,000 employees (up from the few hundred who have access today).
Want to learn more about Incorta’s real-time, no-data-warehouse analytics technology? Contact me directly at osama.elkady@incorta.com.