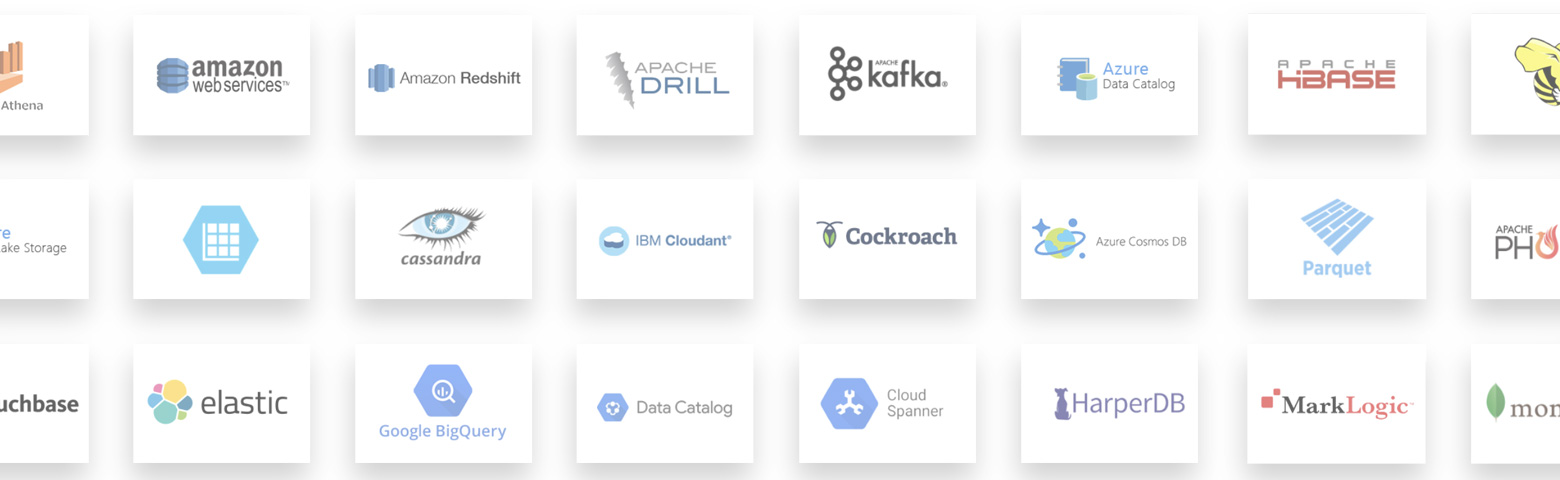
Is yesterday’s data good enough for you?
If you said yes, you can stop reading.
If the answer is no, you’ll want to check out our EVP of Product Strategy Matthew Halliday’s recent conversation with information management analyst and author, William McKnight, on The Bloor Group’s Briefing Room webcast:
The topic was modernizing data architectures, with an emphasis on rethinking data pipelines to support machine learning, artificial intelligence, and real-time analytics.
McKnight explains that architectures are becoming more complex by necessity as more machine learning and artificial intelligence is added to the equation. Against this backdrop, organizations must take advantage of composable parts – like prebuilt extractors and other tools to speed and simplify processes and enhance the capabilities of the core stack – in order to keep it from bogging down under the weight of new demands.
Halliday brings it to life by demonstrating how Incorta’s Intelligent Ingest product solves some of these problems:
“Analytics Ready” 10x Faster
With Intelligent Ingest, you can take data from source systems such as Oracle EBS, Oracle Fusion, Oracle Cloud ERP, NetSuite, JD Edwards, and SAP and prepare the data for analytics 10x faster than you can with any other approach. That’s because Intelligent Ingest pulls data into your analytics system(s) in exactly the same shape as it exists in the source system – no transformations required.
This radically simplifies architectural requirements and allows for fast incremental refreshes and sub-second query times. It also means that a large number of queries can be run on a daily basis with few engineers required to support them. With automated data transformation, app innovators can go from raw data to report in a matter of minutes – regardless of where the data exists.
Finding ways to simplify data pipelines is critical because the architectural choices today are overwhelming: there are data lakes, lake houses, enterprise data warehouses (EDWs), unified data analytics platforms (UDAPs) – not to mention data fabric, data mesh, data hubs, and on it goes.
The Art of the Possible
Modern data architecture can become complicated fast. Keep in mind that every organization’s data environment is going to be different because nobody is starting with a blank slate. What’s clear is that rebuilding ERP systems and moving analytics to the cloud without addressing cumbersome data transformation processes only leads to more issues and challenges.
Many organizations are patching up old data pipelines and ETL processes because they can be hard to remove and replace. At the same time, band-aids can only get you so far. Is data critical to the future of your business? If so, then so are your data pipelines.
As Halliday explains, rethinking data pipelines doesn’t just save you money and headaches – it also opens the door to entirely new possibilities, and therein lies the beauty and power of innovation.
“Good Enough” Is Not Good Enough
Today, speed and agility are everything in analytics – yet there are still companies relying almost entirely on legacy data pipelines that were conceived in an on-premises world. That’s how you end up with extremely complex ETL scripts, with batch windows running all night long and teams waiting days, or even weeks, for data they need right now.
The good news is there’s a lot of opportunity to remediate current architectures. But, according to McKnight, the architecture decisions organizations make today must be based on the fact that data warehouse and data lake environments are both going to grow tremendously as organizations collect more types and volumes of data from more places than ever before.
While there are a lot of technology choices, ultimately it’s not about technology. It’s about gaining the ability to make better decisions with data faster. That’s the North Star everyone should keep in sight.
Watch the full episode on-demand here.