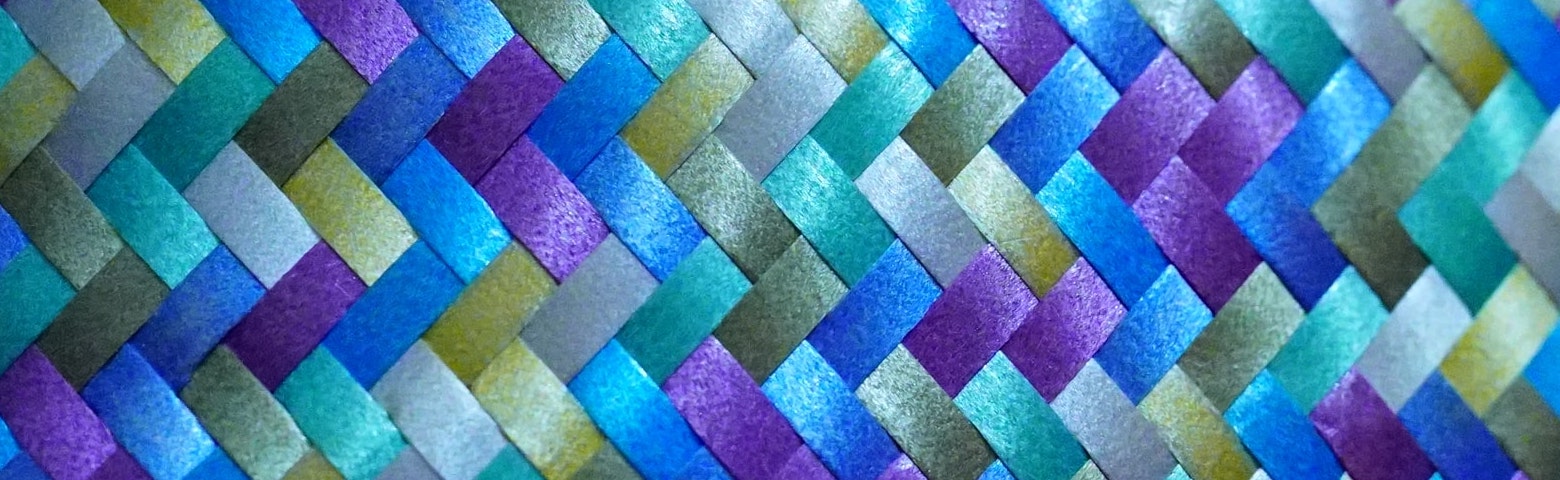
Ever since Gartner began talking about data fabric in 2019 as one of the top trends in data analytics, our sales team has been fielding calls from people looking to buy one.
But we can’t sell them a data fabric. No one can, actually — and that’s because data fabric is not a product. It’s simply a new way to describe something the analytics industry has been chasing for years — the dream of the single enterprise data store, where all data is curated and cataloged and ready for analysis.
The objective of data fabric is to provide a more agile data experience, yielding more timely insights and faster decisions. Simple idea; extremely difficult to pull off.
Why is it trending now? The latest hype builds on the idea that AI can be used to reduce the labor involved in maintaining such a catalog. That’s especially attractive today because there’s growing recognition that traditional workflows and project cycles for data and analytics are out of step with the demands of today’s world.
Every day, more people want more reports and dashboards. As they consume data, they develop new perspectives and have more questions. But while demand for data keeps rising, the supply side of the equation remains tight. There’s only so much data you can push through traditional data pipelines before hitting a complexity barrier.
Something has to change.
The Dream of the Data Fabric
Data fabric, as conceived, would map and connect to all relevant application data stores with metadata to describe data assets and their relationships. Think of it like a browsable data catalog. The data would be composable — meaning that it could be selected, combined, and used in various ways in near real time. You could even run analytics over the metadata to discover insights about the utilization of data.
It’s a great concept, but facts are facts: AI is not up to the task yet.
Some vendors say their solutions are AI-driven, but in reality they are based on rules or heuristics. Cataloging in advance without AI is a non-starter because it’s so much work and the business value is unclear. It’s like doing an archeological dig and cataloging all of the artifacts, not knowing which ones are of any value or interest.
According to Gartner, “No existing stand-alone solution can facilitate a full-fledged data fabric architecture. D&A leaders can ensure a formidable data fabric architecture using a blend of built and bought solutions.”
Build As You Go
The cost-benefit equation of cataloging everything only makes sense if AI can reduce all the manual work. Without it, the only reasonable thing to do is build as you go in response to actual business requirements.
As it stands today, you are better off going with a solution that actually provides value right now and aligns conceptually with the idea of the data fabric. The big concepts are (1) to get all the data in one place, either physically or virtually, without having to know ahead of time what questions you want to answer, and (2) do non-destructive (metadata driven) data preparation so you don’t lose data lineage and detail.
Think of it as a data lake plus catalog — you build up the catalog and metadata over time as you build schemas, views, metrics, and dashboards to address actual business needs.
Directionally Correct
The data fabric concept steps away from the data warehouse paradigm, which forces you to predefine the scope of your analytical inquiries and spend months on data discovery and pipeline development. Instead it points you in the direction you need to go — access to detailed application data, ready to be loaded into a high-performance analytics engine on demand. This makes the business more agile, with an analytics pipeline ready and available to answer whatever question comes to mind.
Right now, the idea that anyone will ever have all their data perfectly organized and cataloged and described is a pipe dream. One day AI may actually make this possible, but the best thing you can do right now is to leverage data fabric concepts as you find business problems and solve them.