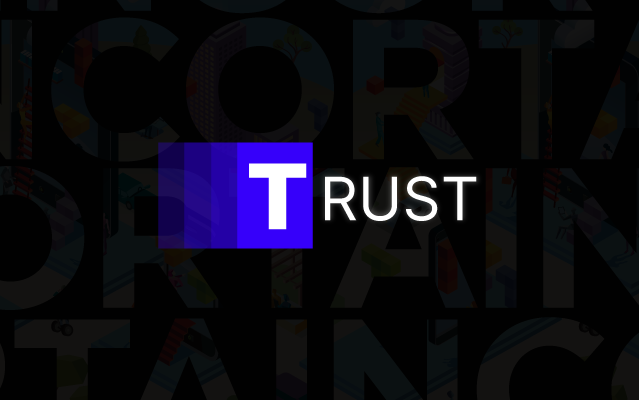
In the middle of the pandemic when everyone was social distancing and staycationing, I decided to spend a few days camping in the mountains of West Virginia. I was shopping on Amazon for a new tent of solid quality, reasonable value and most importantly field tested. I use a very sophisticated methodology for shopping online. I look at the number of reviews, ideally 100+, then add up the percentage of 4-star and 5-star reviews. Products with 85% or better get my attention. Also, products with >10% of 1-star reviews don’t make the shortlist. I told you. Sophisticated.
I found one tent that really fit the bill. It was the right size, the right price, in stock, Prime eligible, and had over 1,000 customer reviews over the years where 85% of people had given either 4 or 5 stars. What stood out was the 12% of 1-star reviews. It seemed like a solid tent. Why so many 1-stars? My gut said it’s probably some kind of a defect or design flaw. A zipper, anchor, rod or similar that leaves people with a bad camping experience. Out of curiosity, I started reading the negative reviews to see if I can find a common thread among different buyers’ experiences. After reading nearly 100 reviews from the previous 12 months, it became clear that most disappointed buyers were not dissatisfied with the product, but because they got their tents four to six weeks after they ordered it. Obviously, the pandemic had dealt a big blow to the supply chain, but many buyers had unfairly punished the product with a 1-star review out of frustration over delivery times. If I backed out of these negative reviews for delivery, the percentage of 1-star ratings would be in the low single digits.
We rely on data for decisions big and small. This is true for business too. So why is it that sometimes we don’t trust what the data is telling us?
Topline metrics and aggregations don’t tell you the whole story
Every enterprise has reports and KPIs (key performance indicators) they track to measure the health of business operations. The data feeding these reports come from ERP systems, business applications and various databases. Unfortunately, this data is also often deconstructed, heavily transformed, and aggregated to create business-ready, consumable datasets. This process results in the loss of much of the original source data and limits how deep you can run your analysis and find usable answers. If you can’t drill into the data and investigate why a metric is outside of benchmarks or expectations, find root cause explanations or isolate anomalies, it’s easy to get misguided. This is when people fall back to guesswork and gut feel and trust in data erodes. Had I accepted the aggregated percentage of 1-star reviews, I would’ve ignored this product and moved on to another.
The data doesn’t agree with you
We rely on data to not only give us answers but also to validate or dismiss our hypotheses and assumptions. There are times when the data completely disagrees with expectations. The kneejerk reaction is to question the accuracy of the analysis or dismiss it altogether. And while the data was correctly analyzed, it will come down to the visibility of the data behind the data to provide the veracity and accuracy that will align expectations and reality. In some cases, it makes sense to combine siloed data from multiple applications to create a more enriched dataset. Using data from multiple sources makes it much easier to cross-check and validate results, increasing the trustworthiness of insights and building alignment and consensus. My kneejerk reaction was that a product defect was to blame for the poor customer reviews.
It just takes too long to get the data
A significant number of decisions are not factored into a multiyear, strategic enterprise business plan. There may be dependencies or decisions that are time-sensitive and need the latest data, modeled, verified and available for analysis. If the data delivery lead times exceed the decision window, the data is not so valuable anymore. When this happens, decisions are made with partial or incomplete data. It’s not as bad as no data, but negatively impacts confidence in the decision outcomes.
Imagine if the detailed reviews were not readily available on Amazon, but if customers can request it at checkout, they get them in the mail in a few days. Now the purchase becomes a gamble, and you may have to send it back or find another product. So much for a hassle-free shopping experience. The speed of data delivery makes an enormous difference in guiding urgent, time-critical decisions and eliminating workflow bottlenecks.
Trust is a foundational, indispensable element to building credibility between the relationship people have with data and the business insights that inform them. As you think about your enterprise data strategy, consider a combination of completeness, transparency, consistency, and timely delivery accompanied with context and rich visualizations. This transforms doubt in data to trust in data. Incorta works with the world’s top enterprises to simplify data access from complex sources including Oracle, SAP and Salesforce and make it instantly available to users and downstream systems. With the latest data in hand and visibility to underlying details, organizations can extract more value from their business data and benefit from confident, trusted and informed decisions.
Ps. It is an exceptional tent for the casual camper.
This is the fourth and final part of our FAST series. If you missed the first three parts, you can find them here: Freshness, Accelerate and Scale.
For more information on trust, check out this customer story from Broadcom