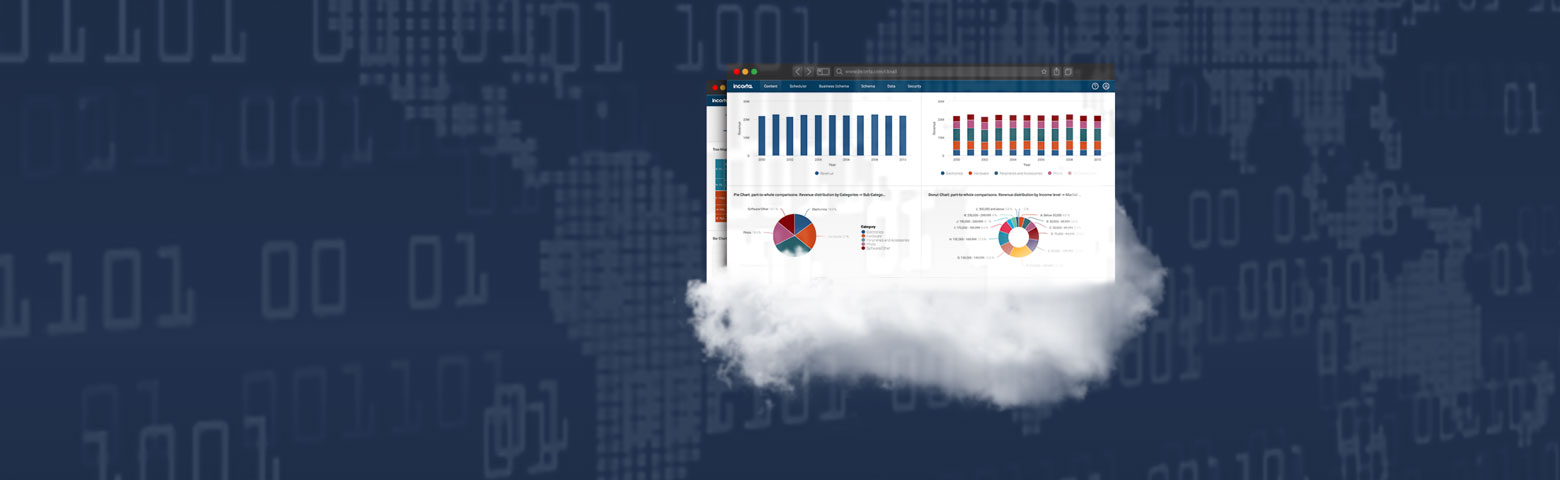
The benefits of cloud computing are widely understood and accepted these days, but organizations are still in the early stages of actually moving systems, applications, and workloads to the cloud. Concerns about security and privacy, along with the sometimes significant upfront effort/investment that must be put forward have caused many organizations to proceed with caution.
If you’re starting to think about how to proceed, or if you’ve already started but are trying to figure out the next step in what is likely an ongoing journey, it’s important to consider that there is significantly more benefit in moving certain types of workloads to the cloud than others. For instance, consider “System A” and “System B” in the chart below:
These “systems” are oversimplified for the purposes of discussion, but System B would be a superior candidate for cloud deployment. Let’s look at why:
Cloud consideration 1: Usage pattern
For a system that has consistent usage patterns, such as System A, the compute resources (mostly CPU cycles and memory) needed on the server(s) that run it are also consistent. On the other hand, if the usage of a system spikes during certain hours of the day or times of the month, as is the case with System B, then a traditional server model would require enough resources to be allocated so that the system can operate effectively at times of peak demand. The rest of the time though, those resources are just sitting there going to waste.
In the cloud, on the other hand, there are ways to deploy applications and systems so that they pull only the resources they need at a particular moment in time—and you only pay for the resources actually consumed. (There are many variables around this in practice, but this general concept is true of any cloud deployment.)
Cloud consideration 2: Compute resources used
The extent to which available compute resources are utilized in a traditional on-premise or data center server model is not only a function of the system usage pattern but also how much CPU power and memory are used in the course of running the application—particularly during its peak usage times. As mentioned above, this is the determining factor of sizing a server in an on-premise deployment model.
As an example: a web gateway that just passes through requests to an application server probably doesn’t burn through much CPU or memory, so it doesn’t need a very powerful server. There is not a large amount of wasted capacity because there isn’t much capacity there to begin with. On the other hand, a server hosting an enterprise data warehouse that is fielding hundreds of complex queries an hour during peak usage would need to be quite beefy! During times of lighter usage, there could be huge amounts of memory and CPU cycles that are going to waste.
Cloud consideration 3: Business criticality
Systems and applications that are essential to the business generally need special architecture to ensure they are up and available anytime the business is operating. This generally involves creating redundancy (having multiple servers that serve the same purpose to eliminate single points of failure) and disaster recovery (having servers available in waiting in a different geographic location, just in case there is a disaster that destroys the regular production system.)
In a properly architected cloud system, redundancy and disaster recovery become far more cost-effective. Instead of purchasing extra hardware upfront that you hope to never have to use, you just pay for the extra capacity if you need it. Furthermore, the core functionality of cloud providers such as AWS is to have data centers in various geographic locations around the globe.
Putting the pieces together
So let’s put meaningful labels on our example systems above. Let’s call “System A” the “Corporate Intranet.” Often intranet sites serve up largely static content and then provide hyperlinks to mission-critical business applications. Sure, being able to access company policies and training materials is useful, but if it’s down for a few hours during a workday the company won’t grind to a halt.
Let’s say “System B” is an “Analytics System.” We’ll say this analytics system includes both reporting and data visualization/exploration tools such as Cognos Analytics or Power BI, as well as data sources such as Incorta, a data lake, and a data warehouse. This system does some pretty heavy lifting for the business—perhaps customer history and trends are being referenced throughout the day by front-line employees. On top of that, data loads and transformations are taking place nightly to populate the latest data from operational systems. And then during each month and year-end closing period, there is a massive amount of financial data being analyzed.
This “Analytics System” is an ideal use case for the cloud. The cloud offers potentially massive amounts of CPU and memory that can be put to use for data transformation, calculation of financial formulas, prediction of future trends, etc. The need for this kind of power is going to be high during certain business hours to serve employees on the front lines, but there will also be spikes during off hours when automated data preparation is taking place, and a huge load put on the system at month and year-end by the office of finance. Finally, all this data is mission critical. It’s being used in both an operational capacity, as well as for tactical and strategic decision making. The unavailability of this data for any period of time could have serious consequences for the business.
While all sorts of systems, applications, and workloads can benefit from being moved to a cloud provider, your data and analytics systems are without a doubt a great place to start—or to tackle next if you’re already on the cloud journey.
This article appeared on the PMsquare Blog and has been published here with permission.
Explore more about how Incorta Cloud can give you a world-class analytics infrastructure in minutes: