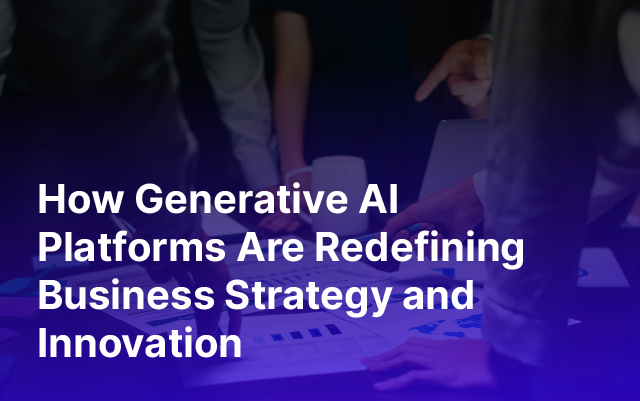
The advent of Generative AI (GenAI) platforms has significantly lowered the barrier to entry for business users looking to adopt AI technologies within their operations. These platforms have made it remarkably easier for non-technical users to leverage the power of AI. By providing access to pre-built models, customizable templates, intuitive interfaces, and drag-and-drop functionalities, GenAI platforms enable business professionals to generate insights, automate tasks, and create content without the need for deep programming knowledge or AI expertise.
This democratization of AI technology means that businesses across industries can now explore innovative solutions and optimize their processes more efficiently than ever before. As a result, GenAI is not just a tool for data scientists and AI researchers but has become an accessible and powerful asset for business users. The broader adoption of AI across the corporate landscape is driving transformative changes in how businesses operate and compete. This emerging technology heralds a new age of human efficiency and effectiveness, with its full potential and societal impact still being unraveled.
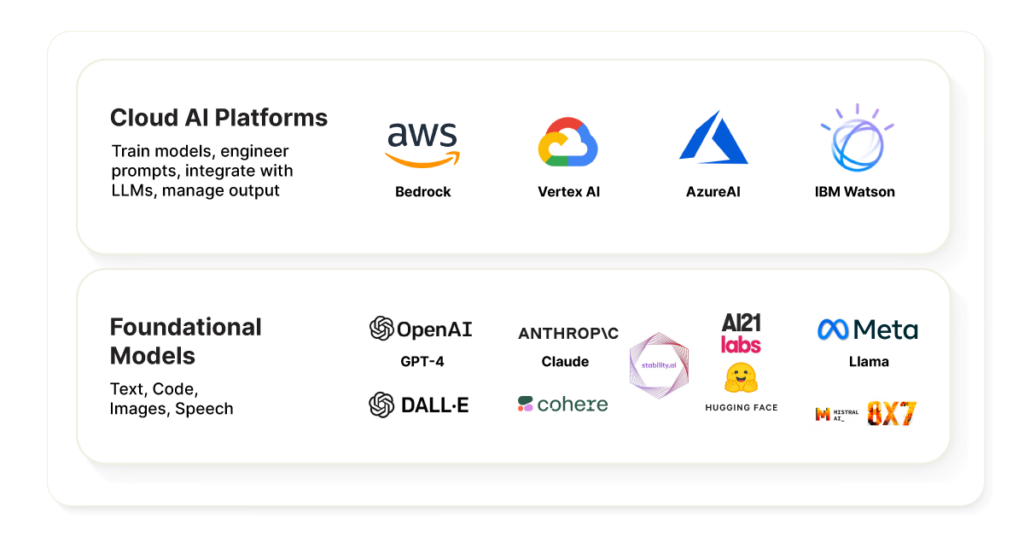
Figure 1: Cloud AI platforms and foundational models.
Public fascination with GenAI has grown through its applications in creative tasks, such as drafting humorous content or visualizing imaginative scenarios like astronauts riding horses. However, for business leaders & enterprise data, the emphasis is on employing GenAI in economically beneficial ways.
To effectively implement GenAI, businesses are turning to advanced AI platforms that offer comprehensive control over GenAI foundational models and their derivatives. These platforms as shown in figure [1] and the foundational models provide the necessary tools to extract insights from enterprise data, encompassing various levels of engagement:
- Level 1: Prompting & Chaining – These platforms offer ready templates and notebooks, allowing users to generate content or chain different AI functions together easily.
- Use Case: A marketing firm uses a GenAI platform to create engaging content. They utilize ready templates of prompts for generating initial drafts of ad copy. Then, leveraging the chaining feature, they seamlessly integrate these drafts with image generation tools to create visually appealing advertisements. This approach saves time and enhances creativity by allowing the firm to rapidly prototype and iterate on marketing materials.
- Level 2: Retrieval-Augmented Generation (RAG) with Managed Embedding & Vector Databases – This enhances search and discovery capabilities, streamlining the process of finding relevant information.
- Use Case: A legal research company employs a GenAI platform with RAG capabilities to streamline its information retrieval process. By using managed embedding and vector databases to calculate the distance between the transformed text into numbers, the platform can quickly sift through vast archives of legal documents to find relevant case laws and precedents. This significantly reduces the time lawyers spend on research, allowing them to focus more on case strategy and analysis.
- Level 3: Finetuning Pre-Trained Models – Businesses can finetune existing models with their own data stored in systems like Delta Lake, tailoring the AI to their specific needs.
- Use Case: A multinational manufacturing company utilizes GenAI to finetune pre-trained models for enhancing its supply chain operations. The company has a vast amount of data on supplier performance, logistics, and inventory levels stored in Delta Lake. By fine-tuning an AI model with this specific dataset, the company can predict potential supply chain disruptions, optimize inventory levels, and improve delivery times.
- Level 4: Pre-Training from Scratch – Some GenAI platforms claim their cost-effectiveness is an order of magnitude better than competitors in training a foundational model from scratch, offering affordable options to build custom models from the ground up.
- Use Case: A startup specializing in financial forecasting develops a custom GenAI model from scratch using a cost-effective platform. The startup’s unique approach to financial data requires a model that isn’t available off-the-shelf. By pre-training a model from the ground up, they create an AI system that is finely tuned to their unique methodology, providing more accurate forecasts than generic models and giving them a competitive edge in the market.
These comprehensive GenAI levels are becoming an integral part of the corporate strategy. To fully leverage these technologies, leaders must incorporate GenAI into existing workflows, transforming them into an embedded part of customer and employee experiences.
Reflecting on the evolution from initial Large Language Model (LLM) applications to the current shift towards versatile, platform-centric models, it’s evident that GenAI is becoming more sophisticated and integral to business strategies. As businesses continue to explore the potential of GenAI, it’s clear that its integration into existing organizational structures is not just a technological upgrade but a strategic transformation. This transformation requires a dedicated approach, a willingness to experiment, and visionary leadership to ensure that GenAI’s potential is fully realized in solving real-world problems and driving innovation.
As organizations navigate this journey, the ones that can quickly adapt and effectively integrate GenAI into their strategies will be the ones to gain a significant competitive advantage in the rapidly evolving business landscape.
Learn how Incorta continues to push the boundaries of what’s possible in the realm of analytics and data intelligence in our recent webinar. Watch here.